- Dangerous Android apps you should delete from your smartphone right away. Beware, there are.
- Explore and run machine learning code with Kaggle Notebooks Using data from Google Play Store Apps.
Google announced a cleaner and whiter redesign of the Play Store following its Material design language. It introduces a new navigation bar at the bottom that separates games, apps, movies and TV, and books into dedicated tabs for easier browsing. For information on subscription refunds, see returns and refunds on Google Play. Pause a subscription. Some apps will also let you pause your subscription. When you pause a subscription, your subscription will pause at the end of your current billing period. On your Android phone or tablet, open the Google Play Store. If you are serious about getting rid of junk files, then Avast is the best cleaner app for Android in 2021. Play Store Rating – 4.7 Downloads – Over 50 million. Special Features of Avast Cleanup.
Google has started rolling out the latest major Play Store redesign with a cleaner layout. The rollout has been underway for a few weeks now, but Google has made it official now. This signals that the redesign should start showing up for most Android users now.
The new visual refresh to the Play Store makes it look a lot cleaner than before, with a lot of white space that makes it easier to identify the content. The old design had a green bar on the top that took the focus away from the content.
The company said on its official blog, “Aligning with Material design language, we’re introducing several user-facing updates to deliver a cleaner, more premium store that improves app discovery and accessibility for our diverse set of users.”
The other highlight of the new Play Store design is the introduction of a new navigation bar at the bottom. This navigation bar divides the Play Store in four categories, namely – Games, Apps, Movies & TV and Books. The default category that loads when you open the Play Store is Apps.
Here are the highlights of the Play Store redesign:
- There are now two distinct destinations for games and apps, which helps us better serve users the right kind of content.
- Once users find the right app or game, the updated store listing page layout surfaces richer app information at the top of each page.
- This makes it easier for users to see the important details and make a decision to install your app.
Apart from this, Google has also mandated developers to update their app icons to meet the new requirements, which state that the icon should be in the shape of a rounded square.
This post attempts to give readers a practical example of how to clean a dataset. The data we wrangle with today is named Google Play Store Apps, which is a simply-formatted CSV-table with each row representing an application.
Dataset Name: Google Play Store Apps
Dataset Source: Kaggle
Task: Data cleaning
Language: Python
Column description
Overall, there are 13 columns:
- App: Application name.
- Category: Category the app belongs to.
- Rating: Overall user rating of the app (as when scraped).
- Reviews: Number of user reviews for the app (as when scraped).
- Size: Size of the app (as when scraped).
- Installs: Number of user downloads/installs for the app (as when scraped).
- Type: Paid or Free.
- Price: Price of the app (as when scraped).
- Content Rating: Age group the app is targeted at – Children / Mature 21+ / Adult.
- Genres: An app can belong to multiple genres (apart from its main category). For eg, a musical family game will belong to Music, Game, Family genres.
- Last Updated: Date when the app was last updated on Play Store (as when scraped).
- Current Ver: Current version of the app available on Play Store (as when scraped).
- Android Ver: Min required Android version (as when scraped).
(copied from the data source.)
Data Cleaning
Load and take an overview
In general, to have an overview of the data frame, I would print out the following information:
- data shape: the number of instances and features.
- several data rows: to have a sense of the values each data point may contain.
- data types of the columns.
- common statistics of the data frame (using .describe() method)
- Missing-value status (using .isna() method).
- The number of unique values for each column.
Remove duplicates
The above shows that we have 10841 rows, however, only 9660 of their App names are unique. This raises a question: Do the duplicated names refer to the same app or not?
If the Play Store restricts the app’s names that different apps must have different names, those duplicates in the dataset are duplicated data points and should be handled so that only 1 of the duplicates remains.
However, it turns out that Google does allow apps with exactly the same name, except for the names that were declared as trademarks, in which case, the names will be protected by the laws. However, we have no clue if any names from these applications have been registered as a trademark, so, to maintain data integrity, we assume that every name in this dataset is duplicatable (i.e. many apps may have the same name).
In other words, we should not delete a row just because its app name is identical to another row. Nevertheless, it is unrealistic to think that 2 different apps may be the same in every property, from the name, rating, reviews to size, etc. So, we remove the duplicates that coincide with another one in all the listed features.
Column: Last Updated
This should obviously be a Date Time column, yet, pandas recognizes it as of object type (the default data type). This implies there is an issue with this column, let’s fix it.
A simple effort to cast this column to datetime gets the following error:
There seem to be some rows with ill-formatted values. The below code will show those rows out.
The problem is clear: in row 10472, there is a missing for the value at the Category. To solve this, we shift all the values of this row to the right:
On a side note, shifting the values of this row to right also solves the problem you may have noticed at the beginning of this work, that the maximum value of the Rating is perceived to be 19.0.
Now, how about the category of this app? Well, I do a search on the Play Store and take it that this app belongs to the LIFESTYLE group. Hoping that this app’s category hasn’t changed from 11 Feb 2018, we fill it in:
Ok, so we can convert the Last Updated column into datetime without any errors.
Column: Rating
The rating is already in the form of a float-like string, we only need to make a call to convert it.
Column: Reviews
Similarly, the Reviews column is also ready to be cast to the Integer type.
Column: Size
Amongst all the apps in this dataset, none has its size reach 1GB. All the values we have to parse either end with ‘M’ (Megabyte), ‘k’ (kilobyte) or equal to ‘Varies with device’. The below script verifies that all of our 10358 rows fall into 1 of these 3 options.
When converting these values into float, we must have them in the same unit, either MB or kB is fine. In this notebook, I choose to use the Megabyte unit, thus each app’s size that was originally in kB will be divided by 1024.
The last problem is: How to handle the ‘Varies with device‘ value. Technically, this is not so much different from NaN as we have no information about the actual size of the application. However, the take is we know that these apps have different versions available at once.
To conclude, we set the rows with ‘Varies with device‘ NaN and make a new dummy column named Variable Size to better distinguish them.
Column: Installs
The number of installs is shown by buckets. Let’s see which buckets are there:
The smallest values are ‘0’, ‘0+’, and ‘1+’. This is quite surprising to me that both ‘0’ and ‘0+’ exist even though we need only one of them. After a bit of searching on the internet, I couldn’t find the official source of Play Store about these bucket counts. However, as stated by most other sources, ‘x+’ has the lower bound as x+1. That is, ’50+’ means 51-100, ‘100+’ means 101-500, and so on. Thus, it makes sense to deduce that ‘0’ means 0, ‘0+’ means 1, ‘1+’ means 2-5, etc. (Please correct me if I’m wrong.)
Follow the above rule, we convert this column to Integer type:
Column: Type
Apart from Free and Paid, this column also has 1 NaN, which is shown below:
Notice that this is also the only row with 0 Install, which makes me feel there might be something wrong with this record.
Further investigation shows that this game seems to appear on Play Store later than the recorded time in the dataset. Wikipedia says it was first on Play Store from 2018-12-04, which is after the Last Updated value 2018-06-28.
Because of this ambiguity, I decide to remove this row from the data.
This column, Type, is then replaced by ‘Is Free’ – a dummy variable.
Column: Price
The price of an app seems to follow this rule: ‘0’ if it is free, else a dollar sign followed by a floating-point number. Let’s check if there are any exceptions:
There is no exception. Great. We can go straight to the conversion code:
Note that although this Price column does cover the information of the Is Free column, I decide to keep both of them for now to emphasize the difference between free and paid apps. This use of dummy variables is elaborated in the post about when should we add a dummy variable.
Later, in case we are concerned with multicollinearity or similar issues, we would remove Is Free if necessary.
Column: Content Rating
Let’s take a look at all the content rating tags:
These tags seem to follow the standard of America.
We have 2 problems with this column: How should Unrated be understood and How to handle this ordinal variable.
Play Store Cache Cleaner
- Unrated apps will not be shown when doing content filtering. If you set up parental controls to restrict apps and games to a certain rating, you won’t see any Unrated apps in the Play Store. Given that in mind, should we treat Unrated apps as NaN or a number larger than 18?
To have a clearer view of the situation, let’s check the distribution of the values:
There are only 2 unrated apps in the dataset. As the number is so small, we have the option to remove them if we think doing so will not bias our data mining process.
For now, I decide to keep these rows to not affect the completeness of the dataset. ‘Unrated’ will be converted to NaN in the following step.
- The Content Rating is obviously an ordinal variable. And Ordinal variables can be either transformed into categorical or interval variables. In this case, which one should we choose?
A detailed tutorial on how to treat ordinal variables is given in another post. In short, for practical purposes, ordinal variables are recommended to be converted to interval in most cases. We will stick with this for now.
Note that aside from converting tags to the corresponding ages, we also have the option to convert them to ranking, i.e. ‘Everyone’ is mapped to 0, ‘Everyone 10+’ to 1, ‘Teen’ to 2, etc.
Column: Android Ver
Look at the value counts:
and the missing values:
we have these observations:
- There are 2 NaN values.
- More than a thousand apps are not specific about their version, i.e. ‘Varies with device‘.
- Several apps stopped updating for newer Android versions.
We would:
- Have a column ‘Android Major Ver From‘ that stores the minimum major version of Android each app supports. ‘Varies with device‘ will be converted to NaN in this column.
- Have a dummy column ‘Variable Android Ver‘ that equals to 1 iff its Android Ver is ‘Varies with device‘.
- Have a dummy column ‘Is Still Maintained’ that equals to 1 iff it still supports the latest Android version.
Note that we by removing ‘Android Ver’, we actually lose some information about the minor Android version. It depends on our purposes on mining this dataset that we decide to extract the minor version or not (in most case, the answer seems to be No).
Column: Current Ver
Some information can be extracted from this column are:
- If this is the first version of the app.
- If the app has multiple versions for different devices.
- The current major version. Note that this might be a bit noisy due to the preference of the developers themselves: with the same change, some app-producers might declare a new major version while others think it is more suitable for a minor version.
It is also worth mentioning that many values in this field do not follow the standard. I list some of those values in the table below. We will treat those as NaNs.
Column: Category and Genres
We will convert these 2 columns to numerical with one-hot encoding. It is lucky that there are no NaNs here. However, note that while an app may only belong to 1 category, there might be multiple genres associate with it.
Free drawing tool for mac. Handling the Category is simple:
It involves a bit more work for the Genres: Kannada kadambari serial.
Everything is done. Here are the first 5 rows of the resulting data frame after being cleaned.
What we have done are:
- Load and show some basic statistics of the dataset.
- Check and fix the problem of missing a comma in this CSV-saved dataset (row 10472).
- Re-format data type of columns from string to int or float or datetime accordingly.
- Remove an erroneous row.
- Make several dummy columns to emphasize some traits (Variable Size, Variable Android Ver, Variable App Ver).
- One-hot encode Category and Genres (Genres may contains multiple values per row).
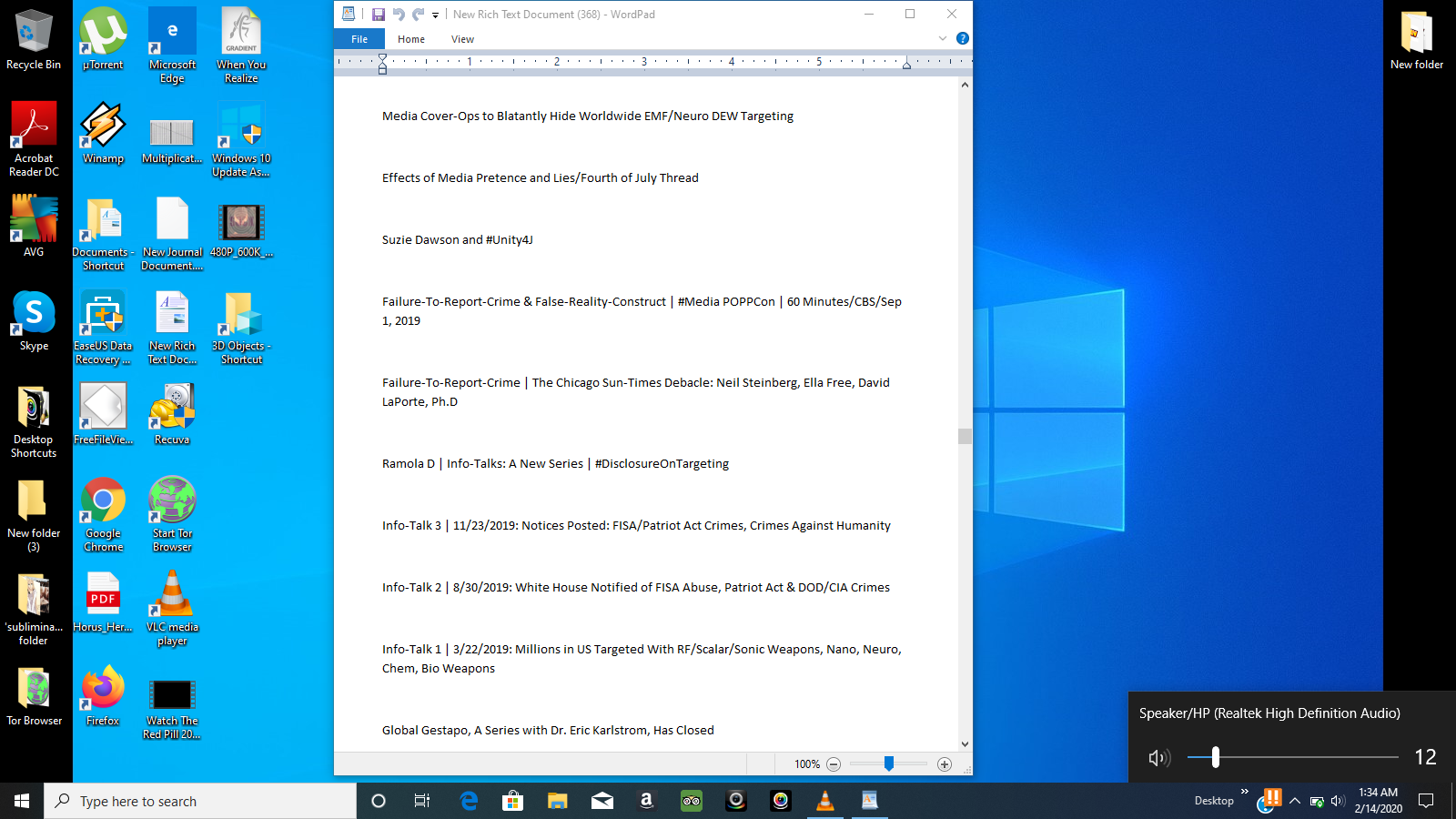
Play Store App
The Jupyter Notebook containing full code is given here.
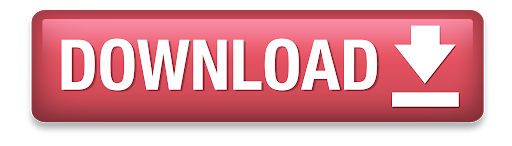